[See my post with Edelman on AI takeover and Aaronson on AI scenarios. This is a rough, with various fine print, caveats, and other discussions missing. Cross-posted on Windows on Theory.]
One challenge for considering the implications of “artificial intelligence,” especially of the “general” variety, is that we don’t have a consensus definition of intelligence. The Oxford Companion to the Mind states that “there seem to be almost as many definitions of intelligence as experts asked to define it.” Indeed, in a recent discussion, Yann LeCun and Yuval Noah Harari offered two different definitions. However, it seems many people agree that:
- Whatever intelligence is, more computational power or cognitive capacity (e.g., a more complex or larger neural network, a species with a larger brain) leads to more of it.
- Whatever intelligence is, the more of it one has, the more one can impact one’s environment.
1 and 2 together can already lead to growing concerns now that we are building artificial systems that every year are more powerful than the last. Yudkowski presents potential progress on intelligence with something like the following chart (taken from Muehlhauser):
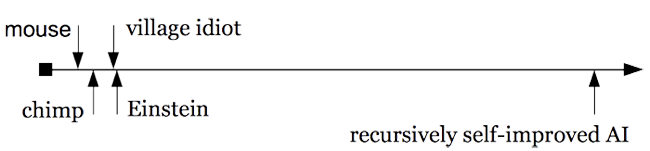
Given that recent progress on AI was achieved by scaling ever larger amounts of computation and data, we might expect a cartoon that looks more like the following:
(Don’t take this cartoon or numbers too seriously. It is obtained by superimposing a hypothetical 1000T param model on the figure from Bolhuis, Tattersall, Chomsky and Berwick. 100T connections in Homo sapiens brain is a rough estimate. Axes implicitly assume synaptic density scales with volume.)
Whether the first or the second cartoon is more accurate, the idea of constructing intelligence that surpasses ours to an increasing degree and on a growing number of dimensions is understandably unsettling to many people. (Especially given that none of the other species of the genus Homo in the chart above survived.) This post is not to say that we should not worry about this. Instead I suggest a different metaphor for how we could think of future powerful models.
Whose intelligence is it?
In our own species’ evolution, as we have become more intelligent, we have become more able to act as agents that do not follow pre-ordained goals but rather choose our own. So we might imagine that there is some monotone “agency vs. intelligence” curve along the following:
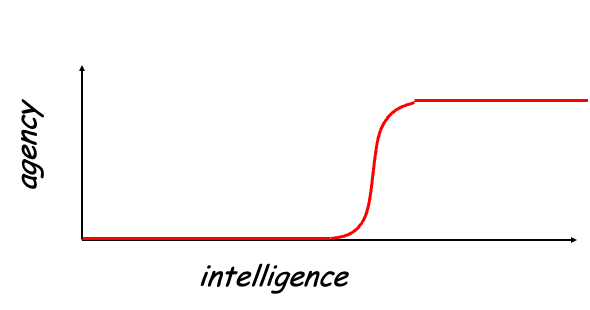
(Once again, don’t take the cartoon too seriously; whether it is a step function, sigmoid-like, or some other monotone curve can be debatable and also depends on what one’s definitions of “agency” and “intelligence” are.)
But perhaps intelligence does not have to go hand-in-hand with agency. Consider the property of physical strength. Like intelligence, this is a capability that an individual can use to shape their environment. I am (much) weaker than Olga Liashchuk, who can lift a 300kg Yoke and walk 24 meters with it in under 20 seconds. However, if I were to drive a forklift, the combination of me and the forklift would be stronger than her. Thus, if we measure strength in functional terms (what we can do with it) instead of by artificial competitions, it makes sense to consider strength as a property of a system rather than an individual. Strength can be aggregated to combine several systems into a stronger one or split up to use different parts of the capacity for different tasks.
Is there an “intelligence forklift”? It is hard to imagine a system that is more intelligent than humans but lacks agency. More accurately, up until recently, it would have been hard to imagine such a system. However, with generative pretrained transformers (GPTs), we have systems that have the potential to be just that. Even though recent GPTs undergo some adaptation and fine-tuning, the vast majority of the computational resources invested into GPTs is used to make them solve the task of finding a continuation of a sequence given its prefix.
We can phrase many general problems as special cases of the task above. (Indeed, with multimodal models, such tasks include essentially any problem that can be asked and answered using any type of digital representation.) Hence as GPT-n becomes better at this task, it is arguably becoming arbitrarily intelligent. However, it is still not an agent but rather a generic problem-solver. In that sense, GPTs can best be modeled as intelligence forklifts.
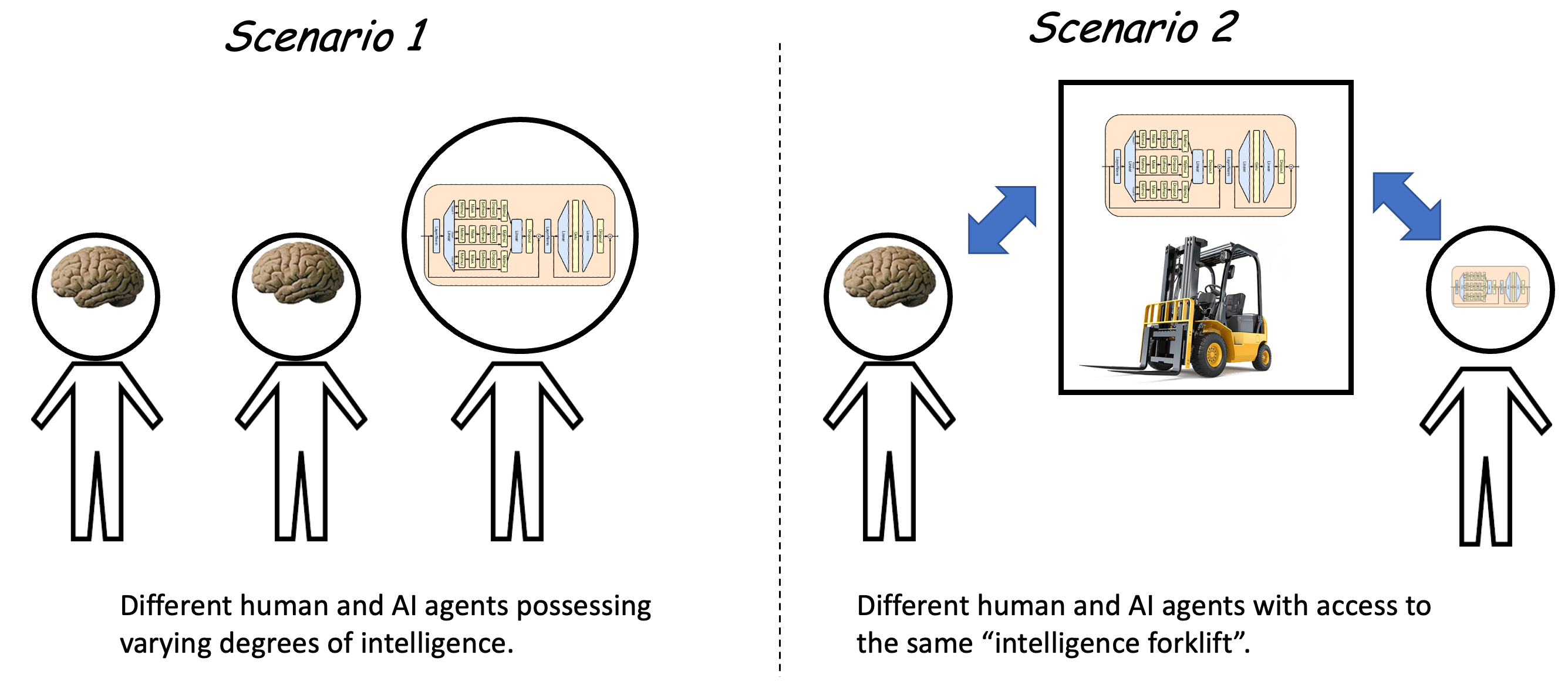
By “intelligence forklift” I mean that such a model can augment an agent with arbitrary intelligence to complete the goals the agent seeks. The agent may be human, but it can also be an AI itself. For example, it might be obtained using fine-tuning, reinforcement learning, or prompt-engineering on GPT. (So, while GPT is not an agent, it can “play one on TV” if asked to do so in its prompt.) Therefore, the above does not mean that we should not be concerned about an artificial highly intelligent agent. However, if the vast majority of an agent’s intelligence is derived from the non-agentic “forklift” (which can be used by many other agents as well), then a multipolar scenario of many agents of competing objectives is more likely than a unipolar one of a single dominating actor. The multipolar scenario might not be safer, but it is different.
Of course, the forklift still has its limitations. Certainly you need to learn how to drive and operate it or you could end up damaging whatever you are trying to lift or move. They can break down and have costly repairs. People have injured and crushed in forklift rollovers.
“The Occupational Safety & Health Administration estimates that forklifts account for 61,800 minor injuries, 34,900 serious injuries, and 85 forklift related deaths every year”.
I hope AI is not too much like a forklift.